Performance of Machine Learning and AI in the Healthcare Sector
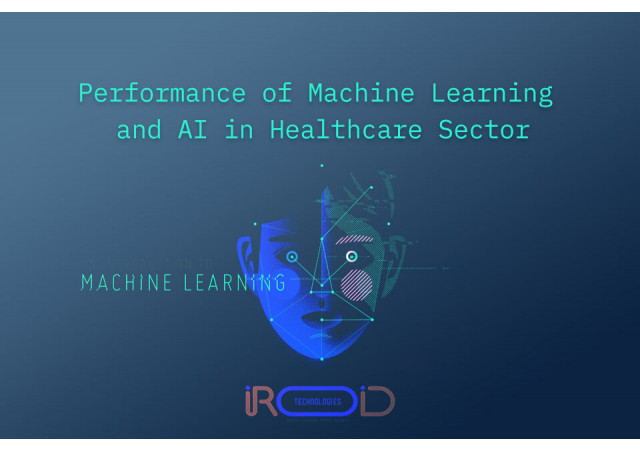
Artificial intelligence (AI) and similar technologies are generally believed in business and community and are running to be employed in healthcare. These technologies have the potential to modify many features of patient care, as well as administrative methods within the provider, payer, and pharmaceutical companies.
There are now several research materials hinting that AI can perform as well as or more reliable than humans at key healthcare duties, such as diagnosing illness. Today, algorithms are previously defeating radiologists at detecting malignant tumors, and guiding researchers in how to build bands for costly clinical cases. However, for a change of reasons, we understand that it will be many years before AI replaces people for comprehensive medical method domains. In this article, we represent both the potential that AI allows to automate characters of care and some of the restrictions to the expedited implementation of AI in healthcare.
Types of AI of importance to healthcare
Artificial intelligence is not one technology, but preferably a collection of them. Most of these technologies have direct relevance to the healthcare profession, but the special processes and jobs they sustain differ broadly. Some particular AI technologies of high rank to healthcare are explained and detailed below.
Neural networks and deep learning
Machine learning is a mathematical system for fitting models to data and to ‘learn’ by practicing models with data. Machine learning and AI in the Healthcare Sector is one of the various common modes of AI; in a 2018 Deloitte survey of 1,100 US directors whose companies were already attempting AI, 63% of companies studied were employing machine learning in their professions. It is a broad technique at the essence of many paths to AI and there are many variants of it.
Natural language processing
Making knowledge of the human language has remained a goal of AI researchers considering the 1950s. This track, NLP, includes applying such as speech perception, text analysis, interpretation, and other goals compared to language. There are two fundamental strategies to it: statistical and semantic NLP. Statistical NLP is based on machine learning (deep learning neural networks in particular) and has committed to a fresh increase in the efficiency of recognition. It requires a comprehensive ‘corpus’ or body of language from which to discover.
Also Read Software Development Myths you should be aware of
Rule-based expert systems
Expert systems based on models of if-then rules were the advancing technology for AI in the 1980s. They were largely used commercially in that and more contemporary periods. In healthcare, they were universally employed for ‘clinical judgment support’ expectations over the last couple of decades and are still in broad use today. Many automated health records (EHR) providers provide a set of rules with their operations today.
Physical robots
Physical robots are properly understood by this period, given that more than 200,000 industrial robots are offered each year throughout the globe. They execute predefined duties like lifting, repositioning, welding, or collecting objects in places like factories and elevators and delivering types of equipment in clinics. More newly, robots have become more collaborative with people and are more readily trained by walking them in the wanted task. They are also shifting to be more responsible, as other AI abilities are being installed in their ‘brains’ (especially their operating systems). Over time, it appears likely that the same advances in intelligence that we've seen in other regions of Machine Learning and AI in the healthcare sector would be organized into solid robots.
Robotic process automation
This technology gives structured digital tasks for organizational purposes, i.e. those concerning information systems as if they were an individual user following a scenario or rules. Related to other forms of AI they are cheap, easy to perform, and apparent in their actions. Robotic process automation (RPA) doesn't really affect robots – only computer programs on servers. It relies on a mixture of workflow, business exercises, and a ‘presentation layer’ combination with data practices to move like a semi-intelligent user of the systems. In healthcare, they are used for repeated tasks like previous authorization, updating inmate records, or billing. When coupled with other technologies like vision recognition, they can be used to obtain data from, for example, faxed images to input them into transactional operations.
Diagnosis and treatment purposes
Diagnosis and treatment of illness have been a locus of AI since at most trivial the 1970s when MYCIN was developed at Stanford for diagnosing blood-borne bacterial diseases. This and other old rule-based systems conferred promise for correctly diagnosing and managing disease, but were not selected for clinical usage. They were not examined more stable than human diagnosticians, and they were inadequately combined with clinician workflows and other medical record systems.
Patient engagement and adherence applications
Patient commitment and adherence have long been seen as the ‘last mile obstacle of healthcare – the final stop between useless and good health results. The more cases proactively compete in their own well-being and care, the greater the outcomes – utilization, economic outcomes, and member participation. These factors are frequently being approached by big data and AI.
Administrative applications
There are also large common administrative purposes in healthcare. The use of Machine Learning and AI in the healthcare sector is slightly less potentially fatal in this domain as correlated to patient care, but it can present substantial powers. These are needed in healthcare because, for example, the common US nurse spends 25% of work time on administrative and organizational activities. The technology that is most expected to be connected to this purpose is RPA. It can be practiced for a variety of applications in healthcare, including applications processing, clinical documentation, income cycle management, and pharmaceutical records management.
On concluding with Machine Learning and AI in the healthcare sector
We believe that AI has an essential role to play in the healthcare presents of the future. In the application of machine learning and AI in the Healthcare Sector, it is the first ability behind the development of correctness medicine, widely accepted to be a very demanded improvement in care. Although primary efforts at sponsoring diagnosis and treatment instructions have proven exciting, we assume that AI will ultimately realize that domain as well. Given the accelerated advances in AI for imaging analysis, it seems likely that most radiology and pathology images will be considered at some point by a computer. Speech and text recognition are previously applied for tasks like patient communication and acquisition of clinical notes, and their practice will increase. To take your step into ML and AI get professional guidance with iROID Technologies, the top software development company in India.
Take a look at our recent article: Looking for the most recent software development trends in 2022? Here we go!